|
Gwenaël Doërr - Technicolor R&D, France
Checking Up the Health of Multimedia Security
|
Short Biography:
Gwenaël Doërr (M’06–SM’12) received the M.Sc. degree in telecommunications systems from Telecom Sud-Paris, Evry, France, in 2001, and the Ph.D. degree in signal and image processing from the Université de Nice Sophia-Antipolis, Nice, France, in 2005. He was a Lecturer of Digital Rights Management with the Department of Computer Science, University College London, London, U.K., from 2005 to 2009. In Spring 2008, he was a Visiting Scholar with HP Labs, Palo Alto, CA, USA, to work on the interoperability of DRM systems. In 2010, he joined the Security and Content Protection Labs, Technicolor Research and Development France, Cesson-Sévigné, France, as a Senior Research Scientist on content protection. His research interests encompass various aspects of multimedia security technologies. His recent works focused on signal processing techniques for antipiracy, including transactional watermarking for different types of content, content fingerprinting for resynchronization, and passive forensics analysis to characterize pirate samples, and piracy channels. Dr. Doërr is currently the Chair of the IEEE Signal Processing Society Technical Committee on Information Forensics and Security. He is also a Distinguished Member of Technicolor’s Fellow Network. He is an Associate Editor for the IEEE TRANSACTIONS ON INFORMATION FORENSICS AND SECURITY and the EURASIP Journal on Image and Video Processing and an Area Editor for the IEEE SIGNAL PROCESSING MAGAZINE. He co-organized Information Hiding in 2007 in St Malo, France, and the IEEE Workshop on Information Forensics and Security in 2009 in London, U.K.
|
Abstract:
The terminology "multimedia security" gained a new popularity in the mid-90s with the rapid rise of digital watermarking in an attempt to combat piracy of copyrighted content. This milestone incarnates the mutation of content protection techniques from conventional cryptography to signal processing techniques. Today, multimedia security encompasses a much wider range of techniques such as multimedia encryption, content fingerprinting, anti-camcording, passive forensic analysis. These various techniques share a common feature, namely operating in the presence of an adversary who aims at interfering with the nominal behavior of the system. In this talk, we will survey 20 years of research in multimedia security and highlight enduring technical challenges that remain to be solved.
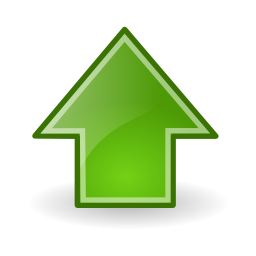
|
Shin'ichi Satoh, NII, Tokyo
Observing Society via Television - Challenges towards Social Analysis by Using Large-Scale Broadcast Video Archive
Short Biography:
Shin'ichi Satoh is a professor at National Institute of Informatics (NII), Tokyo. He received PhD degree in 1992 at the University of Tokyo. His research interests include image processing, video content analysis and multimedia database. Currently he is leading the video processing project at NII, addressing video analysis, indexing, retrieval, and mining for broadcasted video archives.
|
Abstract:
We can obtain many interesting aspects only by watching television, e.g., what's going on in Japan and the world, what is the current trends, how is economic activities, and so on. This talk will introduce couple of trials to automatically analyse such information by computers. Especially, with NII TV-RECS video archive containing 300,000 hours of broadcast videos, we developed and deployed couple of key technologies including face detection and matching, fast commercial film mining, and visual object retrieval towards social analysis tools.
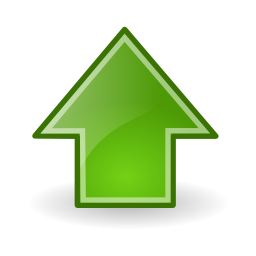
|
Milos Radovanovic, Assistant Professor at the Department of Mathematics and Informatics, Faculty of Sciences, University of Novi Sad, Serbia
Hubs in Nearest-neighbor graphs : Origins, Applications and Challenges - Click on the photo to open the file [time: 1h: 23mn]
Abstract:
The tendency of k-nearest neighbor graphs constructed from tabular data using some distance measure to contain hubs, i.e. points with in-degree much higher than expected, has drawn a fair amount of attention in recent years due to the observed impact on techniques used in many application domains. This talk will be organized into three parts:
(1) Origins, which will discuss the causes of the emergence of hubs (and their low in-degree counterparts, the anti-hubs), and their relationships with dimensionality, neighbourhood size, distance concentration, and the notion of centrality;
(2) Applications, where we will present some notable effects of (anti-)hubs on techniques for machine learning, data mining and information retrieval, identify two different approaches to handling hubs adopted by researchers – through fighting or embracing their existence – and review techniques and applications belonging to the two groups; and
(3) Challenges, which will discuss work in progress, open problems, and areas with significant opportunities for hub-related research.
The questions to Milos Radovanovic [time: 5:11mn]
The slides (Pdf)
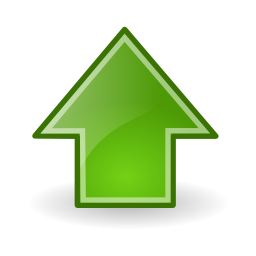
|
Laurent Amsaleg, IRISA (team-project LinkMedia)
A Database Perspective on Large Scale High-Dimensional Indexing - [time: 51: 27mn]
Laurent Amsaleg, researcher at Irisa (team-project LinkMedia), has presented his HDR defence. (Some members of his Jury, Michel Crucianu, Shin'ichi Satoh and Marcel Worring have too presented a talk during the workshop). You can access his talk. |
|
November 21st 2014
|
Michel Crucianu, CEDRIC - CNAM - Paris
Multimedia information retrieval: beyond ranking - Click on the photo to open the file [time: 41:27mn]
Abstract:
Result ranking by assumed relevance was a simple yet powerful idea whose implementation was continuously improved over several decades. The success of search engines returning lists of ranked results shows that this approach does satisfy the users to some extent. However, user needs have a broader spectrum, results can be relevant in different ways to a same query and the structure in the set of results may itself be meaningful.
Ranking alone cannot convey this complexity and becomes a bottleneck in the access to information. Unfortunately, the use of ranked lists is so entrenched that one mechanically attempts to satisfy his information needs by crafting sequences of queries directed to ranking-based search engines.
This talk will question the nature of user needs when searching for multimedia content and explore some emerging solutions, together with problems to be solved.
The questions to Michel Crucianu [time: 5:30mn]
(Note: you might want to increase the sound level for questions in order to
hear microphone-less people in the audience.)
The slides (Pdf)
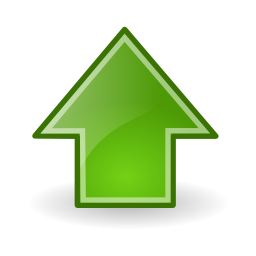
|
Marcel Worring, University of Amsterdam
Multimedia Analytics: synergy between human and machine by visualization - Click on the photo to open the file [time: 40:29mn]
Abstract:
In this talk I present the work of Jan Zahalka, Stevan Rudinac and me on developing multimedia analytics approaches to accessing large image collections. We report on an extensive survey of over eight hundred papers of which hundred papers were identified as being most relevant for the topic. These have been used to develop a novel multimedia analytics model. In the model, the need for semantic navigation of the collection is emphasized and multimedia analytics tasks are placed on the exploration-search axis. The axis is composed of both exploration and search in a certain proportion which changes as the analyst progresses towards insight. Categorization is proposed as a suitable umbrella task realizing the exploration-search axis in the model.
Finally, the pragmatic gap, defined as the difference between the tight machine categorization model and the flexible human categorization model is identified as a crucial multimedia analytics topic. To illustrate the utility of the model we report on a first instantiation in a multi-modal recommender system.
The questions to Marcel Worring [time: 6:54mn]
(Note: you might want to increase the sound level for questions in order to
hear microphone-less people in the audience.)
The slides (Pdf)
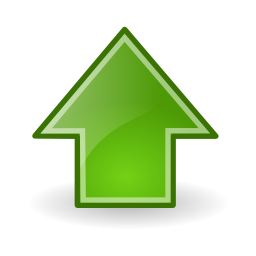
|
Arthur Zimek, LMU Germany
Challenges for Unsupervised Ensemble Learning
Short Biography:
Dr. Arthur Zimek is a Privatdozent in the database systems and data mining group at the Ludwig-Maximilians-Universität München (LMU), Germany. 2012-2013 he was a postdoctoral fellow in the department for Computing Science at the University of Alberta, Edmonton, Canada. He holds degrees in bioinformatics, philosophy, and theology, involving studies at universities in Munich, Mainz (Germany), and Innsbruck (Austria) and finished his Ph.D. thesis in informatics on ''Correlation Clustering'' at LMU in summer 2008. For this work, Zimek received the ''SIGKDD Doctoral Dissertation Award (runner-up)'' in 2009. His research interests include clustering and outlier detection, methods as well as evaluation, and high dimensional data. Zimek published more than 50 papers at peer reviewed conferences and in international journals. Together with his co-authors, he received the ''Best Paper Honorable Mention Award'' at SDM 2008 and the ''Best Demonstration Paper Award'' at SSTD 2011. Zimek has been a member of program committees of the leading data mining conferences (e.g. SIGKDD, ECMLPKDD, CIKM, SDM) and serves as reviewer for journals like ACM TKDD, IEEE TKDE, Data Mining and Knowledge Discovery (Springer), Machine Learning (Springer).
|
Abstract:
We discuss the use of ensemble techniques for unsupervised learning, with a focus on outlier detection. To introduce the field, we will briefly sketch the data mining task of unsupervised outlier detection and discuss some basic considerations about ensemble techniques. Then we give an overview on existing approaches to using ensemble techniques for outlier detection as well as on the challenges in doing so. Some of our recent contributions to this field will be discussed in more detail, highlighting the issue of diversity of models in building effective ensembles. Finally, we will return to the broader perspective and reason about the application of ensemble techniques in the context of unsupervised learning in general.
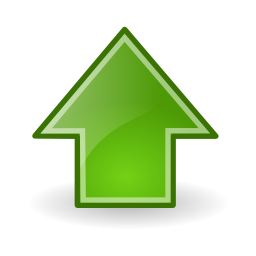
|
Erich Schubert, LMU - Germany
Normalization of Scores and Distances for Ensemble Methods
Short Biography
Erich Schubert is a research and teaching assistant in the database systems and data mining group at the Ludwig-Maximilians-Universität München, Germany. He studied mathematics and computer science and finished his Ph.D. thesis on "Generalized and Efficient Outlier Detection for Spatial, Temporal, and High-Dimensional Data Mining" in 2013. He received the "Best Demonstration Paper Award" at SSTD 2011 for his work on spatial outlier detection together with his co-authors. This tutorial is closely related to his line of research and several of his recent publications.
Abstract:
In classification, ensembles can often be built on a binary decision. But in outlier detection, we need to work with the outlier detection scores, and perform a combination of the numerical values. For this, we need to make the scores of different detectors comparable, which gets difficult if we intend to combine different algorithms, where scores may have a very different meaning. We present a statistical model for interpreting and normalizing outlier detection scores based on robust estimation of distributions and Bayesian reasoning, and touch on numerical challenges arising in this context. We then outline how these normalizations can be put into the different context of data normalization, how distance functions can be modeled as ensembles of primitive similarity measures, and sketch ideas for future work on improving the analysis of high-dimensional data by careful normalization of dissimilarities and distance ensembles designed for high-dimensional data.
|
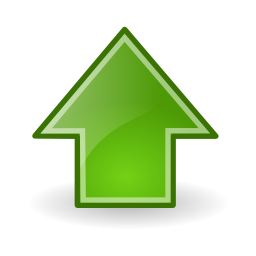
|
|
|